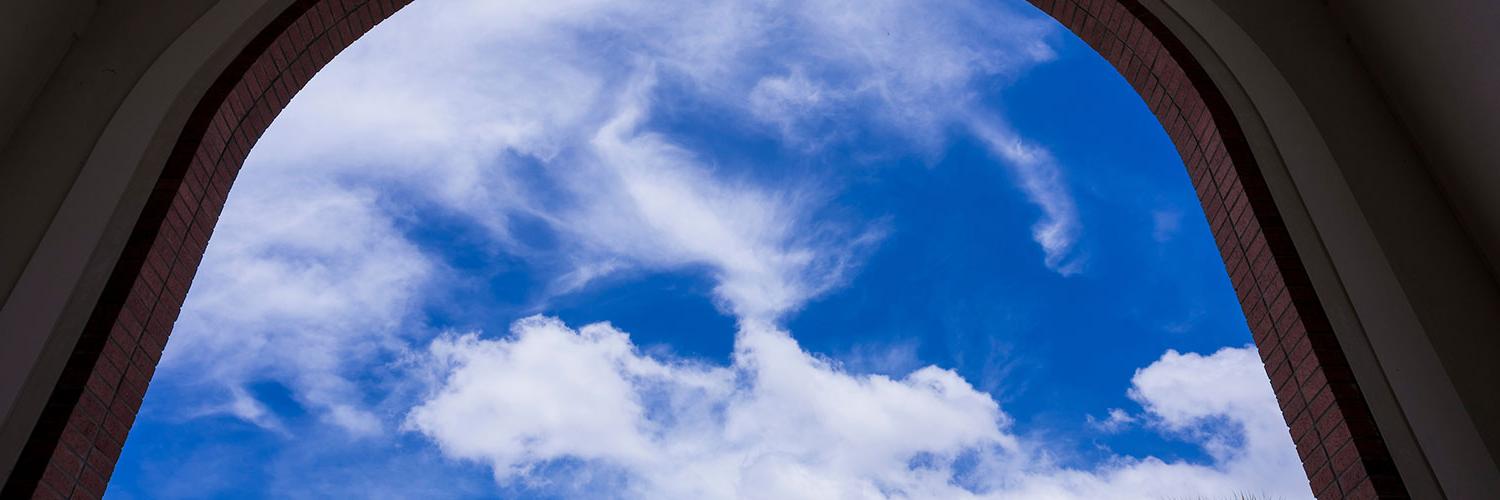
Chan, Philip

Philip Chan
Associate Professor | College of Engineering and Science - Electrical Engineering and Computer Science
Contact Information
pkc@theabsolutelongestwebdomainnameinthewholegoddamnfuckinguniverse.com
(321) 674-7280
Harris Center For Science and Engineering, 209
(321) 674-7280
Harris Center For Science and Engineering, 209
Expertise
machine learning, data mining, anomaly detection, learning from imbalanced data
Educational Background
B.S., Computer Science Southwest Texas State University 1986
M.S., Computer Science Vanderbilt University 1988
Ph.D., Computer Science Columbia University 1996
Professional Experience
Associate Professor, Computer Science, Florida Institute of Technology (2002-present); Assistant Professor (1997-2002); Visiting Assistant Professor (1995-97).
Visiting Scientist/Faculty (sabbatical), Laboratory of Computer Science, Massachusetts Institute of Technology (2002-3).
Selected Publications
- Representation learning with function call graph transformations for malware open set recognition.
J. Jia and P. Chan
Proc. Intl. Joint Conf. Neural Networks, to appear, 2022. - A Machine Learning Approach to Predicting SEP Events Using Properties of Coronal Mass Ejections.
J. Torres, L. Zhao, P. Chan, and M. Zhang
Space Weather, 20(7), 2022. - Learning a Neural-network-based Representation for Open Set Recognition. [supplement]
M. Hassen and P. Chan
Proc. SIAM Intl. Conf. Data Mining, pp. 154-162, 2020. - Estimating Effectiveness of Twitter Messages with a Personalized Machine Learning Approach.
X. Sun and P. Chan.
Knowledge and Information Systems, 56(1):27-53, 2018. - Scalable Function-Call Graph based Malware Classification.
M. Hassen and P. Chan
ACM Conference on Data and Applications Security and Privacy (CODASPY), pp. 239-248, 2017. - Improving Efficiency of Maximizing Spread in the Flow Authority Model for Large Sparse Networks. [longer version]
P. Chan and E. Ahmadzadeh
Proc. IEEE Intl. Conf. Big Data, pp. 863-868, 2016.
Research
- Machine Learning and Data Mining
- Anomaly Detection
- Learning from imbalacned data